Artificial intelligence techniques for the automatic detection of colorectal polyps
DOI:
https://doi.org/10.22516/25007440.471Keywords:
colonoscopy, colorectal cancer, polyps, detection, Artificial IntelligenceAbstract
Colorectal cancer (CRC) is one of the most prevalent malignant tumors worldwide. These neoplasms originate from adenomatous lesions or polyps that must be resected to prevent the development of the disease, and that can be done through a colonoscopy. Polyps are reported during colonoscopy in 40% of men and 30% of women (hyperplastic, adenomatous, serrated, among others), and, on average 25% are adenomatous polyps (the main indicator of quality in colonoscopy). However, these lesions are not easy to visualize because of the multiplicity of blind spots in the colon and human errors associated with the performance of the procedure. Several research works have reported that about 25% of colorectal polyps are overlooked or undetected during colonoscopy, and as a result, the patient may have interval cancer. These figures show the need for a second observer (artificial intelligence system) to reduce the possibility of not detecting polyps and prevent colon cancer as much as possible.
Objective: To create a computational method for the automatic detection of colorectal polyps using artificial intelligence using recorded videos of colonoscopy procedures.
Methodology: Public databases of colorectal polyps and a data collection constructed in a university hospital were used. Initially, all the frames in the videos were normalized to reduce the high variability between databases. Subsequently, polyps were detected using a deep learning method with a convolutional neural network. This network starts with weights learned from millions of natural images taken from the ImageNET database. Network weights are updated using colonoscopy images, following the fine-tuning technique. Finally, polyps are detected by assigning each box a probability of polyp presence and determining the threshold that defines when the polyp is present in a box.
Results: This approach was trained and evaluated with 1 875 cases collected from 5 public databases and the one built in the university hospital, which total approximately 123 046 frames. The results obtained were compared with the markings of different experts in colonoscopy, obtaining 0.77 accuracy, 0.89 sensitivity, 0.71 specificity, and a receiver operating characteristic curve of 0.87.
Conclusion: This method detected polyps in an outstanding way, overcoming the high variability caused by the types of lesions and bowel lumen condition (loops, folds or retractions) and obtaining a very high sensitivity compared with an experienced gastroenterologist. This may help reduce the incidence of human error, as it is one of the main factors that cause polyps to not be detected or overlooked during a colonoscopy.
Downloads
References
Bray F, Ferlay J, Soerjomataram I, Siegel RL, Torre LA, Jemal A. Global cancer statistics 2018: GLOBOCAN estimates of incidence and mortality worldwide for 36 cancers in 185 countries. CA Cancer J Clin. 2018;68(6):394- 424. https://doi.org/10.3322/caac.21492
Data I, Method L. Colombia Estadisticas GLOBOCAN. 2018;380:1-2.
Samadder NJ, Curtin K, Tuohy TMF, Pappas L, Boucher K, Provenzale D, et al. Characteristics of missed or interval colorectal cancer and patient survival: A population-based study. Gastroenterology [Internet]. 2014;146(4):950-60. Available from: http://dx.doi.org/10.1053/j.gastro.2014.01.013
Kaltenbach T, Sano Y, Friedland S, Soetikno R. American Gastroenterological Association (AGA) Institute Technology Assessment on Image-Enhanced Endoscopy. Gastroenterology. 2008;134(1):327-40. https://doi.org/10.1053/j.gastro.2007.10.062
Sr B, Baraza W. Chromoscopy versus conventional endoscopy for the detection of polyps in the colon and rectum ( Review ). 2010;(10).
Paris Workshop ParticipantsThe Paris endoscopic classification of superficial neoplastic lesions: esophagus, stomach and colon. The Paris endoscopic classification of superficial neoplastic lesions: esophagus, stomach, and colon. Gastrointest Endosc. 2003;58(6):S3-43. https://doi.org/10.1016/S0016-5107(03)02159-X
Dinesen L, Chua TJ, Kaffes AJ. Meta-analysis of narrow-band imaging versus conventional colonoscopy for adenoma detection. YMGE [Internet]. 2012;75(3):604-11. Available from: http://dx.doi.org/10.1016/j.gie.2011.10.017
Nagorni A, Bjelakovic G, Petrovic B. Narrow band imaging versus conventional white light colonoscopy for the detection of colorectal polyps ( Review ) Narrow band imaging versus conventional white light colonoscopy for the detection of colorectal polyps. 2012;(1):10-2. https://doi.org/10.1002/14651858.CD008361.pub2
Jin X, Chai T, Shi J, Yang X, Sun Q. Meta-analysis for evaluating the accuracy of endoscopy with narrow band imaging in detecting colorectal adenomas. 2012;27:882-7. https://doi.org/10.1111/j.1440-1746.2011.06987.x
Komeda Y, Suzuki N, Sarah M, Thomas-Gibson S, Vance M, Fraser C, et al. Factors associated with failed polyp retrieval at screening colonoscopy. Gastrointest Endosc [Internet]. 2013;77(3):395-400. Available from: http://dx.doi.org/10.1016/j.gie.2012.10.007
Choi HN, Kim HH, Oh JS, Jang HS, Hwang HS, Kim EY, et al. [Factors influencing the miss rate of polyps in a tandem colonoscopy study]. Korean J Gastroenterol. 2014;64(1):24-30. https://doi.org/10.4166/kjg.2014.64.1.24
Van Rijn JC, Reitsma JB, Stoker J, Bossuyt PM, Van Deventer SJ, Dekker E. Polyp miss rate determined by tandem colonoscopy: A systematic review. Am J Gastroenterol. 2006;101(2):343-50. https://doi.org/10.1111/j.1572-0241.2006.00390.x
Bernal J, Sánchez J, Vilariño F. Towards automatic polyp detection with a polyp appearance model. 2012;45:3166-82. https://doi.org/10.1016/j.patcog.2012.03.002
Shin Y, Balasingham I, Member S. Comparison of Hand - craft Feature based SVM and CNN based Deep Learning Framework for Automatic Polyp Classification. 2017 39th Annu Int Conf IEEE Eng Med Biol Soc. 2017;3277- 80. https://doi.org/10.1109/EMBC.2017.8037556
Urban G, Tripathi P, Alkayali T, Mittal M, Jalali F, Karnes W, et al. Deep Learning Localizes and Identifies Polyps in Real Time with 96% Accuracy in Screening Colonoscopy. Gastroenterology [Internet]. 2018;155(4):1069- 1078.e8. Available from: https://linkinghub.elsevier.com/retrieve/pii/S0016508518346596. https://doi.org/10.1053/j.gastro.2018.06.037
Taha B, Werghi N, Dias J. Automatic Polyp Detection in Endoscopy Videos: A Survey. Biomed Eng (NY) [Internet]. 2017; Available from: http://www.actapress.com/PaperInfo.aspx?paperId=456449. https://doi.org/10.2316/P.2017.852-031
Szegedy C, Vanhoucke V, Ioffe S, Shlens J, Wojna Z. Rethinking the Inception Architecture for Computer Vision. Proc IEEE Comput Soc Conf Comput Vis Pattern Recognit. 2016;2016-Decem:2818-26. https://doi.org/10.1109/CVPR.2016.308
Simonyan K, Zisserman A. Very Deep Convolutional Networks for Large-Scale Image Recognition. 2014;1-14. Available from: http://arxiv.org/abs/1409.1556
Hembury GA, Borovkov V V., Lintuluoto JM, Inoue Y. Deep Residual Learning for Image Recognition Kaiming. Cvpr [Internet]. 2003;32(5):428-9. Available from: http://image-net.org/challenges/LSVRC/2015/ https://doi.org/10.1246/cl.2003.428
Tajbakhsh N, Gurudu SR, Liang J. Automated polyp detection in colonoscopy videos using shape and context information. IEEE Trans Med Imaging [Internet]. 2016 Feb [cited 2017 Feb 13];35(2):630-44. Available from: http://ieeexplore.ieee.org/document/7294676/ https://doi.org/10.1109/TMI.2015.2487997
Bernal J, Sánchez FJ, Fernández-Esparrach G, Gil D, Rodríguez C, Vilariño F. WM-DOVA maps for accurate polyp highlighting in colonoscopy: Validation vs. saliency maps from physicians. Comput Med Imaging Graph. 2015;43:99-111. https://doi.org/10.1016/j.compmedimag.2015.02.007
Silva J, Histace A, Romain O, Dray X, Granado B. Toward embedded detection of polyps in WCE images for early diagnosis of colorectal cancer. Int J Comput Assist Radiol Surg. 2014;9(2):283-93. https://doi.org/10.1007/s11548-013-0926-3
Freedman JS, Harari DY, Bamji ND, Bodian CA, Kornacki S, Cohen LB, et al. The detection of premalignant colon polyps during colonoscopy is stable throughout the workday. Gastrointest Endosc [Internet]. 2011;73(6):1197- 206. Available from: http://dx.doi.org/10.1016/j.gie.2011.01.019
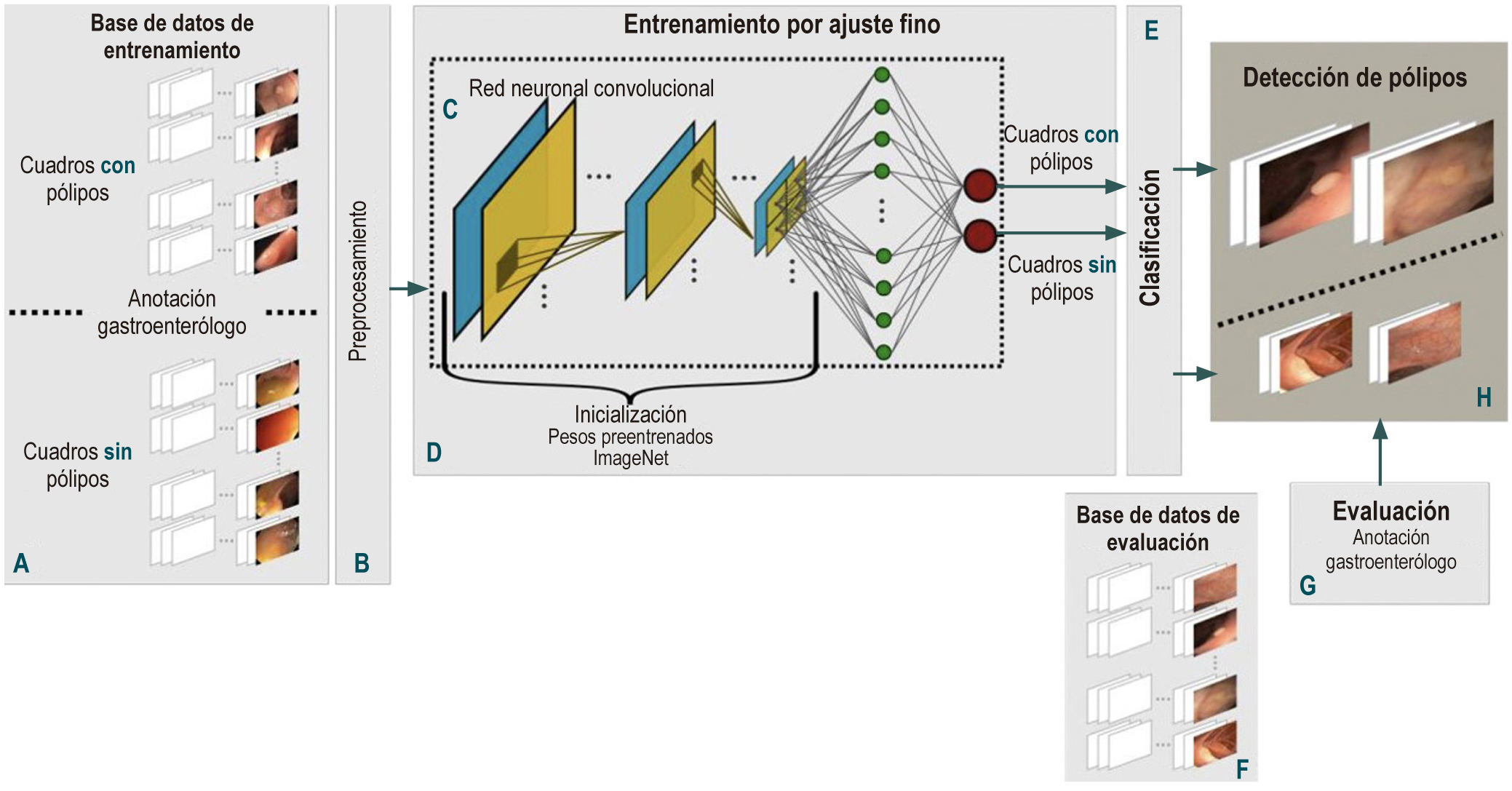
Downloads
Published
How to Cite
Issue
Section
License
Aquellos autores/as que tengan publicaciones con esta revista, aceptan los términos siguientes:
Los autores/as ceden sus derechos de autor y garantizarán a la revista el derecho de primera publicación de su obra, el cuál estará simultáneamente sujeto a la Licencia de reconocimiento de Creative Commons que permite a terceros compartir la obra siempre que se indique su autor y su primera publicación en esta revista.
Los contenidos están protegidos bajo una licencia de Creative Commons Reconocimiento-NoComercial-SinObraDerivada 4.0 Internacional.

Article metrics | |
---|---|
Abstract views | |
Galley vies | |
PDF Views | |
HTML views | |
Other views |